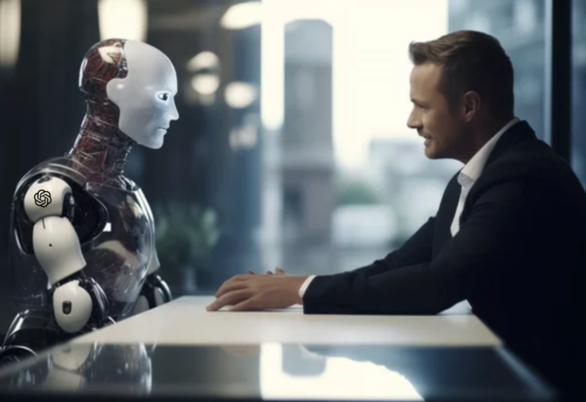
Today, we’re diving into how AI might add to our asbility of predicting crew fatigue risk.
ChatGPT, how do you see AI contributing to the FRM area in a few years from now?
ChatGPT: -AI has fair potential in predicting crew fatigue, beyond what classical statistical methods offer. One of the biggest advantages is AI’s ability to handle vast amounts of data from various sources—like flight schedules, sleep patterns, environmental factors, and even real-time physiological data. By processing this data with machine learning algorithms, AI can identify complex patterns that might not be immediately evident through traditional methods.
That sounds promising. What makes AI more effective than classical statistical approaches?
-The key difference lies in adaptability and complexity. Traditional statistical methods often rely on predefined models and assumptions, which can limit their ability to capture the nuanced relationships between different fatigue factors. AI, particularly machine learning, excels at learning from data itself, without needing explicit instructions on what to look for. This allows AI to continuously improve its predictions as more data becomes available and as conditions change, such as new regulations or changes in flight durations.
Are there any specific AI techniques that would be particularly useful in this context?
-Yes, techniques like deep learning and ensemble learning could be particularly powerful. Deep learning can analyze sequential data, such as changes in roster patterns over longer time frames, which may be of importance for a better understanding how fatigue develops. Ensemble learning, on the other hand, combines multiple models to improve prediction accuracy, which is valuable given the vast variability in roster patterns and differences between individuals.
What are the potential downsides or challenges of relying on AI for fatigue prediction?
-While AI offers many advantages, it also comes with some challenges. One of the main concerns is the “black box” nature of some AI models, particularly deep learning. These models can be very accurate, but it’s often difficult to understand how they arrive at their predictions. This lack of transparency can be a significant drawback in an industry like aviation, where safety and accountability are paramount.
Moreover, AI systems require vast amounts of high-quality data for training. Collecting this data, especially sensitive information like biometric data, raises concerns about privacy and consent. There’s also the challenge of ensuring that AI models remain unbiased and do not inadvertently reflect certain crew groups better than others.
How do you see the integration of AI with classical methods? Can they complement each other?
-Absolutely. The best approach might be a hybrid one, where AI is used to identify patterns and trends, and classical statistical methods are employed to validate these findings and provide a level of interpretability. For instance, AI could flag a potential driver/contributor to fatigue risk, which can then be further analyzed using traditional statistical techniques to confirm its validity and contribution.
This combination leverages the strengths of both approaches—AI’s power in pattern recognition and adaptability, and classical statistics’ rigor and transparency. Together, they could provide a robust system for managing crew fatigue, enhancing both safety and efficiency in aviation.
It seems that the future of fatigue prediction is both complex and promising. Any final thoughts?
-The future of fatigue prediction in aviation will likely hinge on finding the right balance between AI innovation and the established practices of the industry. While AI offers incredible potential, it’s essential to address its challenges head-on—particularly issues of transparency, data privacy, and bias. If these can be managed, AI could significantly enhance our ability to predict and thereby mitigate crew fatigue, ultimately producing even better crew pairings and rosters than we can do today.
Thank you, ChatGPT, for sharing your insights. It’s clear to me that AI has a role to play in the future of fatigue risk management for airline crew.
Comments