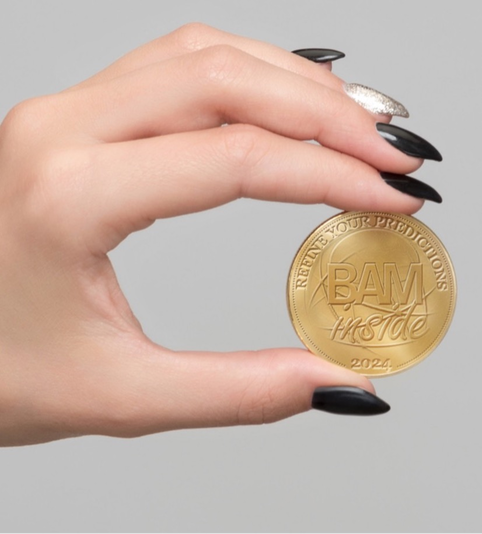
Predictions from a bio-mathematical model are sensitive to certain assumptions about individuals, such as their diurnal type, habitual sleep length (typical amount of sleep per 24 hours), commute time, and the time crew need at home or a hotel for 'winding down' or 'spooling up' before and after sleeping.
Most fatigue models allow users to control these assumptions via parameters, applying the settings to the entire predicted population.
BAM (The Boeing Alertness Model) works differently. These types of assumptions can be controlled per individual and can even be used during crew planning optimization. Normally, it could be quite sensitive to collect the actual diurnal type from crew members, but if they volunteer that information—for example, that they are 'evening types'—the predictions will of course become more accurate. Even if specific information about the crew is not available, better assumptions can be made based on their preferences or age, rather than assuming they are all of 'intermediate type'. For instance, crew members requesting late duties are likely to be inclined towards eveningness. Similarly, crew over 50 years of age are likely to lean towards morningness. Use whatever available information to make improved assumptions, rather than imposing a 'one size fits all' approach.
Commute times in big cities are longer during rush hours, affecting the available sleep opportunity for crew. BAM can reflect different commute times per layover station, even differentiating those based on the time of day. And it doesn't stop there. Learn more about BAM and how it compares to other models in this document.
Comments